8 1 Inference for Two Dependent Samples Matched Pairs Significant Statistics
Table Of Content
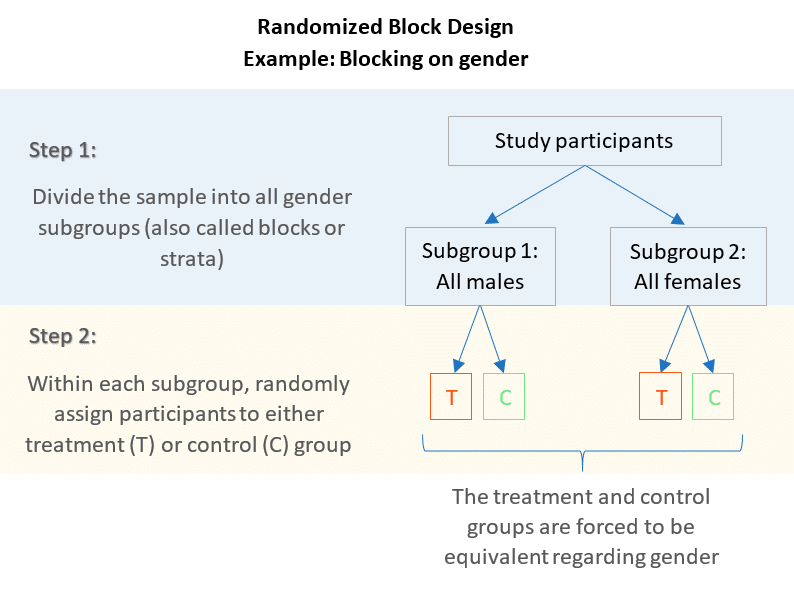
If one subject decides to drop out of the study, you actually lose two subjects since you no longer have a complete pair. Speaking of wallpaper, traditional design is staging a comeback in 2023 — floral print upholstery included. Go all-out with a patterned sofa or accent chair, and take things one step further with an accent print, like a classic stripe. Paired with a solid rug or clean white walls, the look feels elevated and charming. Scale, or the size of the print, should vary between each textile. For example, you can mix a busy leopard print chair with a classic oversized check, or a small floral print with a wide cabana strip.
On how many variables should you match?
In a matched pairs design, treatment options are randomly assigned to pairs of similar participants, whereas in a randomized block design, treatment options are randomly assigned to groups of similar participants. The objective of both is to balance baseline confounding variables by distributing them evenly between the treatment and the control group. Studies that employ smaller sample sizes generally have financial constraints or time constraints, making it unfeasible to have a larger sample size. The matched pairs experimental design is most beneficial for studies that have small sample sizes. This is because it is harder to obtain balanced groups when using small sample sizes, even with the use of random assignment.
Remember: take your time!
Thus, any difference in weight loss that we observe can be attributed to the diet, as opposed to age or gender. Busy, romantic floral patterns need a direct opposite to calm the overall look and achieve balance. Solid colors and graphic, geometric prints always fit the bill — we’re talking romantic blue florals with sleek velvet and classic stripes.
Matched Pairs Experimental Design
Additionally, matched pairs design can only be used when there are two treatment conditions so that one person from each pair can be assigned the first treatment and the other can be assigned the second treatment. No, since a matched pairs design is an experiment, and experimental designs are essentially not susceptible to confounding. Independent measures design, also known as between-groups, is an experimental design where different participants are used in each condition of the independent variable.
Repeated Measures design is an experimental design where the same participants participate in each independent variable condition. This means that each experiment condition includes the same group of participants. It can be quite time-consuming to find subjects who match on certain variables, particularly if you use two or more variables. For example, it might not be hard to find 50 females to use as pairs, but it could be quite hard to find 50 female pairs in which each pair matches exactly on age. There are some notable advantages and some potential disadvantages of using a matched pairs design.
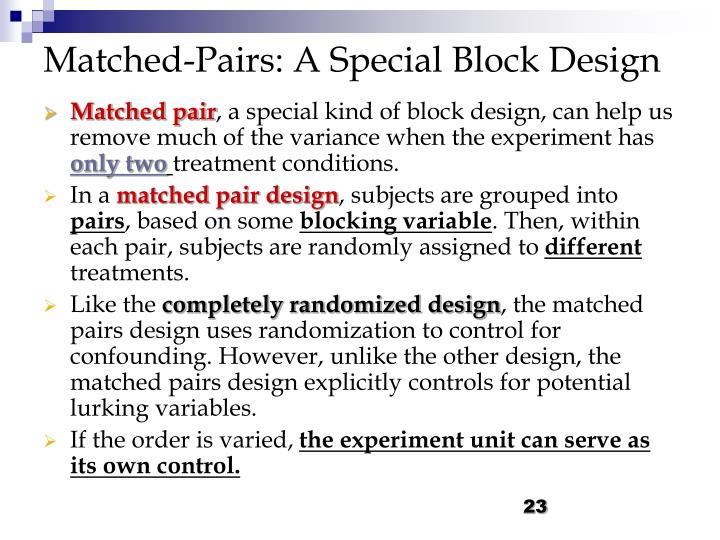
Association between subjective symptoms and obesity and postoperative recurrence in differentiated thyroid cancer: a ... - Nature.com
Association between subjective symptoms and obesity and postoperative recurrence in differentiated thyroid cancer: a ....
Posted: Fri, 28 Feb 2020 08:00:00 GMT [source]
As we will see below in the limitations of pair-matching, if a variable is used as a matching variable, its effect on the outcome can no longer be analyzed in the study. For example, instead of matching a 22-year-old with another 22-year old, researchers may instead create age ranges like 21-25, 26-30, 31-35, etc. so they can match one subject in the age range with another subject in the age range. One way to make it slightly easier to find subjects that match is to use ranges for the variables you’re attempting to match on. A lurking variable is a variable that is not accounted for in an experiment that could potentially affect the outcomes of the experiment. While you can 100% throw caution to the wind and power clash with the best of them, we recommend having at least one color that carries through all patterns — even if it’s not an exact match.
For instance, in order to study the effect of a new sunscreen, the new product can be applied to the right arm (the treatment group), and the left arm can be used as control. Variable(s) that have affected the results (DV), apart from the IV. A confounding variable could be an extraneous variable that has not been controlled. To assess the difference in reading comprehension between 7 and 9-year-olds, a researcher recruited each group from a local primary school. They were given the same passage of text to read and then asked a series of questions to assess their understanding. Although order effects occur for each participant, they balance each other out in the results because they occur equally in both groups.
Pair-matching benefits studies with small samples sizes where it is difficult to obtain balanced groups by complete random allocation. Intentional negative space is absolutely essential when trying to pull off multiple patterns in a single space. It’s arguably just as important as the prints themselves. Always balance printed pieces with clean solids, whether it’s fresh white walls, a solid area rug, or solid throw pillows. For example, a bold patterned sofa might crave solid, textural pillows, and vice versa.
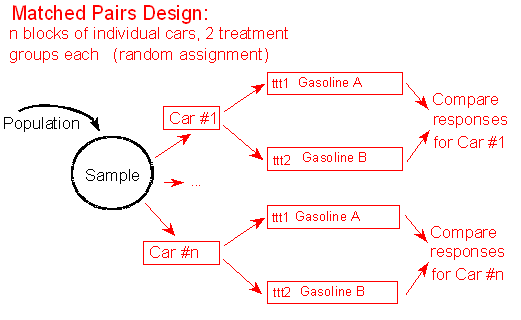
A member will then be allotted to the control group in each pair, and the other member will be assigned to the trial group. The strategies are then equivalent to the free groups’ plan. The mean consequences of the matches would be analyzed after the trial. Picking the wrong matching variables is problematic as it is irreversible. In other words, we CANNOT explore alternative causal hypotheses since the design is definitive and cannot be changed. By improving the comparability of the study participants, matching may also increase the power of the study (the probability of finding an effect when, in fact, there is one).
]Another benefit of matched pairs is their diminished demand attributes. Because we test all members just a single time, members are more averse to figure the analysis’ objective. This might lessen the gamble that members will change a part of their way of behaving because of information on the examination speculation. Therefore, lessening demand attributes might expand the legitimacy of the research. In the past model, both age and orientation can altogether affect weight reduction.
In a perfect world we could assume that both samples come from a normal distribution, therefore the difference in those normal distributions are also normal. However in order to use Z, we must know the population standard deviation which is near impossible for a difference distribution. Also it is very hard to find large numbers of matched pairs so the sampling distribution we typically use for is a t distribution with n – 1 degrees of freedom, where n is the number of differences. Experimental design refers to how participants are allocated to different groups in an experiment. Types of design include repeated measures, independent groups, and matched pairs designs.
This means that each condition of the experiment includes a different group of participants. A study was conducted to investigate how effective a new diet was in lowering cholesterol. Results for the randomly selected subjects are shown in the table. Are the subjects’ cholesterol levels lower on average after the diet?
The obvious pro is that you can find matches more easily, but the con is that the subjects will match less precisely. For example, using the approach above it’s possible for a 21-year-old and a 25-year-old to be matched up, which is a rather notable difference in age. This is a trade-off that researchers must decide is worth or not in order to find pairs more easily. No matter how hard researchers try, there will always be some variation within the subjects in each pair.
You have learned to conduct inference on single means and single proportions. We know that the first step is deciding what type of data we are working with. For quantitative data we are focused on means, while for categorical we are focused on proportions.
Comments
Post a Comment